AI for Brokers and Traders: State-of-the-Art 2022
After over 20 years, the tech industry is wondering if we have any technology left that can revolutionise and evolutionise the brokerage business, or are we stuck in an incremental phase?
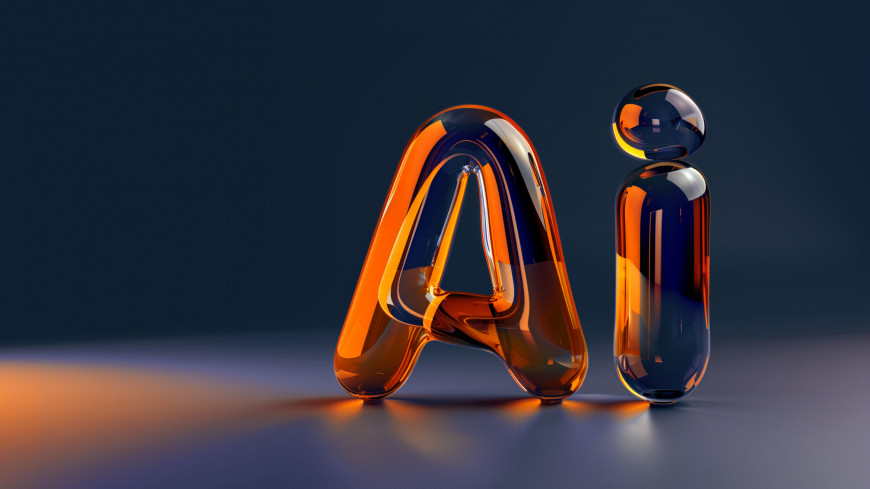
AI and Machine Learning Tools That Traders Look For

The phrase “Artificial Intelligence” is thrown around a lot these days. Typically, the broader the promised set of functions is, the less likely it will actually work.
AI won’t be the “silver bullet” for any given case, but we’d like to shed some light on using it to solve business-oriented problems–specifically trading.
In general, the goal of trading is to make a profit. Its process is tied up with decision-making and technical analysis based on statistical data. The analysis and search for market patterns can be performed by humans, and also by computers and artificial intelligence.
The stock market has been utilising trading robots with a focus on tracing price movements. JPMorgan’s study states that in 2020, over 60% of trades exceeding $10M were executed using algorithms. Furthermore, the algorithmic trading market is expected to grow by $4 billion by 2024, bringing the total volume to $19 billion.
The numbers look significant, but it’s even more important to pay attention to the dynamics.
What makes market players use trading robots and algorithms at an increasing rate? Can AI handle any other functions for different market players?
Let’s look for answers.
Traders’ Expectations

In the past two years, the FX market withstood massive challenges worldwide and experienced growth for several reasons:
- Surge in remote work due to the COVID-19 pandemic, and many people have become unemployed and started looking for a new source of income.
- Increased telecom and internet coverage worldwide has led to a major influx of new traders using a variety of online trading platforms.
- High liquidity of the FX market and low transaction costs made trading, especially through mobile apps, accessible to anyone.
However, those who started dabbling in trading when the pandemic hit decided to step aside in Q3 2021. According to Bloomberg, the total equity volume from individual US investors fell from 24% to 19%. Possibly, this happened due to high market volatility, lack of experience, and the need for time-consuming analysis and market situation monitoring.
If these explanations are in line with the real situation, we can highlight some traders’ needs that brokers can address and make financial markets accessible to a wider audience.
Rapid AI development hasn’t been left unnoticed by retail market participants, either. Old-timers in the financial markets and beginner traders have surely heard a lot about how AI beats humans in solving specific tasks.
We analysed many research papers and use cases in the field of finance and trading, and revealed that today traders expect the following features to be provided by an online brokerage:
- Automation of routine tasks
- Tools that help make more informed decisions
- Risk management
How Brokers Can Meet These Expectations

Let’s discuss how brokers can supply these features to win the race for more customers.
1. Automation of Routine Tasks
Below are the links to several research papers demonstrating that people seek ways to spend less time on data analysis:
Machine Learning-Based Financial Statement Analysis (January 2020)
This paper explores how machine learning is applied to financial statement analysis. It compares a range of machine learning models and their ability to predict abnormal stock returns around earnings announcements. This analysis is based solely on past financial statement data.
Fundamental Analysis of Detailed Financial Data: A Machine Learning Approach (April 2021)
The paper conducts a fundamental analysis of detailed financial information to predict earnings. The authors leverage machine learning methods to combine the high-dimensional financial data into a summary measure that shows significant predictive power.
One of the products that automate data analysis is K Score. It handles large and diverse data sets and runs a variety of predictive models to come up with stock-ranking rating.
2. Tools that Help Make More Informed Decisions—They Lower the Market’s Entry Barrier.
Here are a couple of examples of when AI helps with decision-making:
Alternative data: Quiverquant
“Quiver allows retail investors to tap into the power of big data, and have access to actionable, easy to interpret data that hasn’t already been dissected by Wall Street. [Quiver] scrapes alternative stock data from across the internet and aggregates it in a free, easy-to-use web dashboard.”
Canadian company BUZZ Indexes gathers big data from social media and thematic blogs writing about trading and investments. Then, it uses AI to filter the data and translate it into the online community’s opinion on highly-mentioned stocks. They use 15mln data points to optimise their models to maximize profits.
3. Risk Management
Here’s an example of how AI can exclude human factors for the sake of risk management.
The Aite Group in its report “Hedge Fund Survey, 2020: Algorithmic Trading” argues that the main reason for the growing popularity of trading algorithms is an attempt to reduce the influence of the human factor on the market.
Institutional investors already employ the benefits of algorithmic trading–about 80% of all trading operations on US exchanges are closed using algorithms.
Techtrader is “a fully autonomous stock trading system that requires no human intervention, […]. Requiring no adjustments or updates, it combines a human-like perspective on stocks with the discipline and attention span of a machine.”
What’s In There For Brokers

- Why meet traders’ needs?
- Effects of having and not having AI (“Why now?”)
- How brokers can benefit from AI directly
It’s no secret that clients’ trading activity is one of the main factors that define a broker’s performance. Let’s take a look at how machine learning and AI can help brokers when serving their clients.
ML-based forecasting tools can cover increasing traders’ engagement with trading signals.
Then, a growing number of traders turn to robo-advisors when purchasing and managing their portfolio. Quite opportunely, more research papers have recently discussed using deep neural networks for trading. They suggest that individual investors expect brokers to provide this functionality as assistance when creating a portfolio, so brokerages should start incorporating such tools into their technical stack.
For now, most individual traders manage their portfolios on their own, though brokers can help them select the right instruments with investment advice. However, traders usually wish to know why an instrument is considered profitable at the moment. They’ll also need something else to back it up in addition to the general analytics opinion. In this regard, the solution developed by hoops.ai seems quite intriguing. Brokers can integrate this product with their trading platforms to analyse markets and generate readable and information-rich reports on a selected instrument, so traders can make more informed choices.
When it comes to attracting new traders, lowering the entry barrier for new users might work. A new trader’s user experience depends on the usability and features of a trading platform, but it also counts on the knowledge of the industry and time required for trading.
As a result, a broker obtains a competitive edge by reducing either the required skill level or time spent on trading.
To save essential traders’ time, standalone DL-based trading robots help copy and maintain user-defined strategies.
Production of personalised up-to-date educational content can also be beneficial if it takes into account the latest market events. To make it easier, brokers can leverage generative language model solutions that use AI. That’ll generate unique content based on trader’s interests.
Speaking about generative models, it’s also worthwhile to think about generating marketing content. Brokers could use such models to collect social network opinions and sentiments on the latest trading events and highlight trends. That could even supply the broker’s marketing department with analytical content. It would help the broker’s marketing team save time and effort for other tasks.
Besides content generation, brokers can use ML for semantic analysis of user comments in the company’s social network profile. Support teams can employ such a tool to monitor customer satisfaction and identify the most common issues reported by users. From this feedback, they can teach their models further.
Marketing professionals can also benefit from machine learning by purchasing advertising traffic that is one of the trickiest tasks for the marketing department. The thing is, sometimes you can evaluate the campaign performance only by counting new user LTV over a lengthy time span. Here, data analysis and predictive modelling come to the rescue. Predictive modelling is a mathematical process used to predict future events or outcomes by analysing patterns in a given set of input data.
Trying to attract new users, you shouldn’t forget about keeping the existing ones engaged. Predicting client attrition by trading activity, increased number of support requests, and other factors will help identify the users who aren’t satisfied with the platform or service. Brokers can also keep these users engaged with their platform by sending them automatically generated personalised emails.
Issues that AI and ML Can Solve in Fintech

– Biometrics
– Anti-fraud practice
– AI search for data in corporate systems: defining the essence of search requests and search for documents with vital information
– Reporting
– Virtual assistants for finance planning
– Collecting information from news/social networks for fundamental analysis
– Processing market data/indices for technical analysis
– Consulting on medium-term investment
– Managing and diversifying portfolio
– Comparing analysts and checking their performance when choosing the best strategies for mirror trading
– Building behavioural patterns of market shocks
– Detecting market manipulation and collusion
Human-in-the-Loop vs. Fully Automated AI

Much of what is available on the market comes with significant limitations compared to expectations. If you want to decide on an ML solution that suits your needs, you’ll need to understand the difference between human-in-the-loop and fully-automated technology.
Here are 3 available options:
1. AI Assistance: Provides information for decision-making
Example: ML models can analyse information from multiple different data sources. Then, they’ll provide traders with aggregated results about market sentiment regarding specific instruments. Traders can use obtained information to decide on buying, for example.
2. Partial Automation: Model makes straightforward decisions. Human experts solve the more tricky cases
Example: Customer support chatbots. They help traders find answers to their day-to-day questions. But when they struggle with understanding a user’s intent or have no functionality for resolving the issue, they can transfer the ticket to a human specialist.
3. Full Automation: Human experts don’t intervene in the decision-making process
Example: Reinforcement learning agents for automated stock trading.
Advantages of Automation | Disadvantages of Automation |
More scalable and usually less-time consuming | Prone to inductive bias* |
Decreased human factor | Less control |
*Inductive bias is a set of assumptions that a model has learnt during training and now uses to predict outputs of given inputs that it hasn’t encountered yet.
Risks

As usual, benefits come along with risks. What are the risks of AI in trading?
AI-Based Software Enormously Varies in Quality
AI-advisors and bots are only as good as their underlying software. The bottom line is, they’re manually designed, built, and trained, and people are prone to making mistakes. Your AI bot won’t be worth it if it’s been developed sloppily.
Suspicious Software Vendors
The number of software vendors offering AI-based software is surreal. They promise whole mountains of gold but are yet to deliver on these promises. That’s why, you need to be careful when choosing one.
The Ever-Changing Nature of Markets
As markets constantly change and evolve, you’ll have to update your AI software and bots accordingly. That is, if you plan on making profits from your AI instances in the long run.
Conclusion
Ultimately, we’ve listed many opportunities that AI brings to brokers in terms of engaging and empowering traders and investors. Machine learning technology has come a long way, but it still isn’t perfect. That means brokers willing to tap into the benefits of the technology need to be extra careful when choosing a third-party vendor and deciding to develop some bots without outside help.
Here at Devexperts, we’ve been busy raising our own AI-based virtual assistant, Devexa. It won’t earn you effortless millions but will definitely help to improve the trader’s experience with your brokerage. Devexa will also increase your customer satisfaction rates, and at the same time, reduce the load on your support desk through automation. In a nutshell, our formula for Devexa is advanced machine learning + specific-domain expertise. If you’d like to learn more, head here.
What are the business domains you’d like to automate using artificial intelligence and machine learning? Leave your comments below!